Teaching Computers to Conduct Spoken Interviews: Breaking the Realtime Barrier with Learning
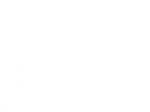
Several challenges remain in the effort to build software capable of conducting realtime dialogue with people. Part of the problem has been a lack of realtime flexibility, especially with regards to turntaking. We have built a system that can adapt its turntaking behavior in natural dialogue, learning to minimize unwanted interruptions and "awkward silences". The system learns this dynamically during the interaction in less than 30 turns, without special training sessions. Here we describe the system and its performance when interacting with people in the role of an interviewer. A prior evaluation of the system included 10 interactions with a single artificial agent (a non-learning version of itself); the new data consists of 10 interaction sessions with 10 different humans. Results show performance to be close to a human's in natural, polite dialogue, with 20% of the turn transitions taking place in under 300 msecs and 60% under 500 msecs. The system works in real-world settings, achieving robust learning in spite of noisy data. The modularity of the architecture gives it significant potential for extensions beyond the interview scenario described here.