GNetIc – Using Bayesian Decision Networks for Iconic Gesture Generation
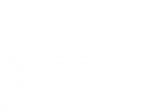
Expressing spatial information with iconic gestures is abundant in human communication and requires to transform a referent representation into resembling gestural form. This task is challenging as the mapping is determined by the visuo-spatial features of the referent, the overall discourse context as well as concomitant speech, and its outcome varies considerably across different speakers. We present a framework, GNetIc, that combines data-driven with model-based techniques to model the generation of iconic gestures with Bayesian decision networks. Drawing on extensive empirical data, we discuss how this method allows for simulating speaker-specific vs. speaker-independent gesture production. Modeling results from a prototype implementation are presented and evaluated.